Thursday, February 20, 2025
Right-Sizing AI Risk management: Smarter Strategies for Effective AI Governance

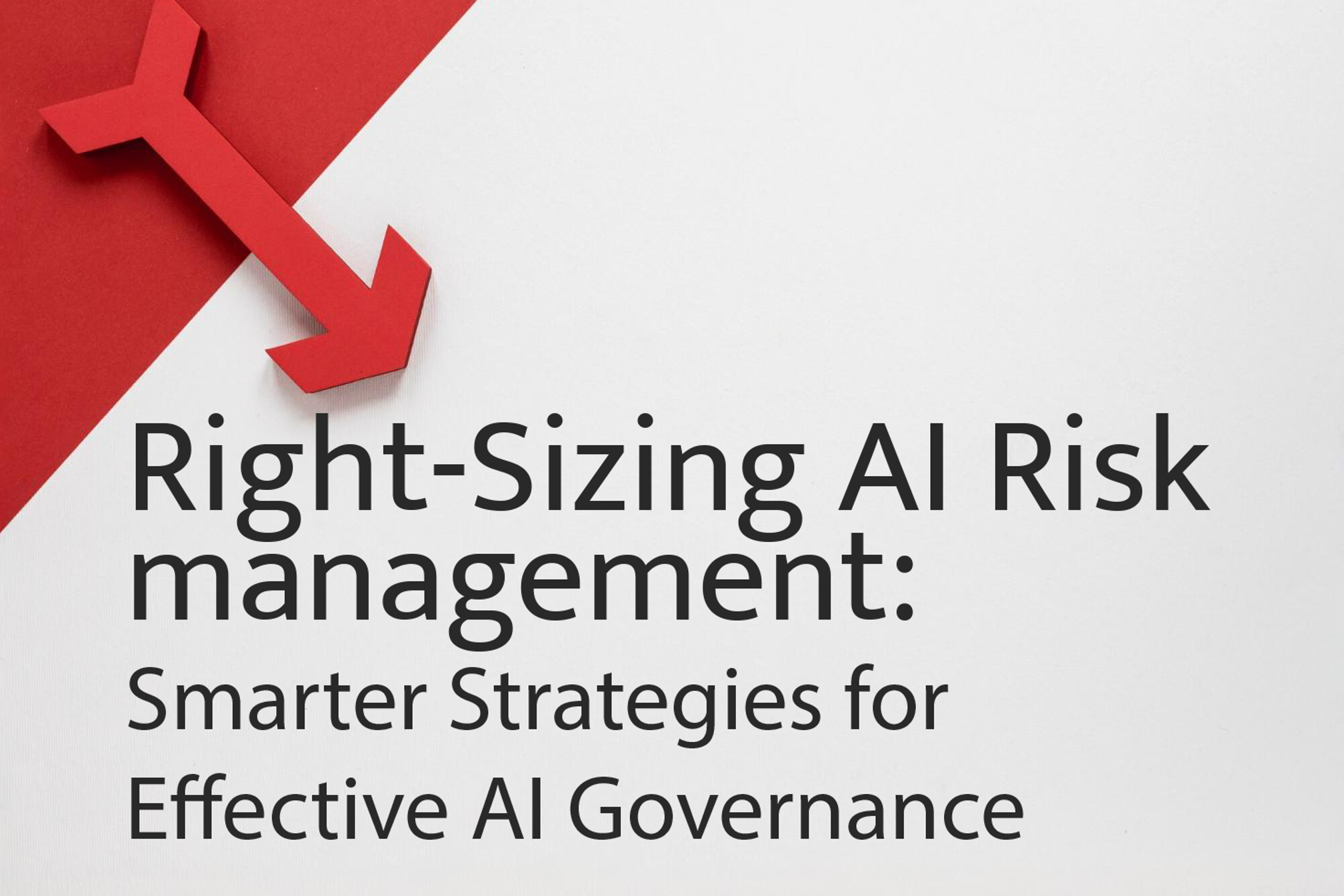
AI Governance frameworks need to be effective, flexible, scalable and comprehensive. Effective to keep risks within tolerance, flexible to cater to new technologies and use cases, scalable so that they can handle a large number and variety of projects without impeding the core business functions of the organization and comprehensive to act as a single reference for risk management of all AI projects. There are inherent tradeoffs within the four characteristics, and navigating them is key to crafting a sustainable AI governance framework.

Importance of Right sizing AI risk management
Right sizing AI risk management helps create scalable and flexible frameworks while maintaining its effectiveness. This is particularly important in the AI context for four reasons:
- The accelerating pace of AI technological change. New algorithms and architectures with novel capabilities and risks are expected to come about with increasing frequency. AI governance frameworks need to be able to address these.
- The rapid commoditization of AI technologies. Third party vendors, libraries and development frameworks are introduced within weeks of new AI algorithms. These trends greatly reduce time to market of new AI applications; coupled with competitive pressures we can expect ever shortening time-to-market cycles for AI driven applications which will place greater demands on governance frameworks to be scalable and not hinder innovation.
- Nature of AI systems and models which require near continuous changes. Whether fine-tuning third party AI models or re-training in-house AI models, AI systems need to be worked on continuously to ensure improvements in performance and alignment.
- The growing application of AI to a wide variety of use cases. Materiality of risk is dependent on a variety of factors including the where and how the AI is applied, the regulatory and legal environment as well as various external circumstances.
A one size fits all approach is difficult to operationalize then given the realities of modern AI systems highlighted above.
Flexibility
Our paper on AI Governance addresses both flexibility and scalability in detail and draws some inspiration from the work by NIST. In particular we have found it effective to create a two tiered structure which separates the different functions of AI governance. By doing so we can ensure effectiveness of the program by ensuring organization-wide policies are respected, while being flexible in following different workstreams for different use cases based on appropriate risk triage/categorization.
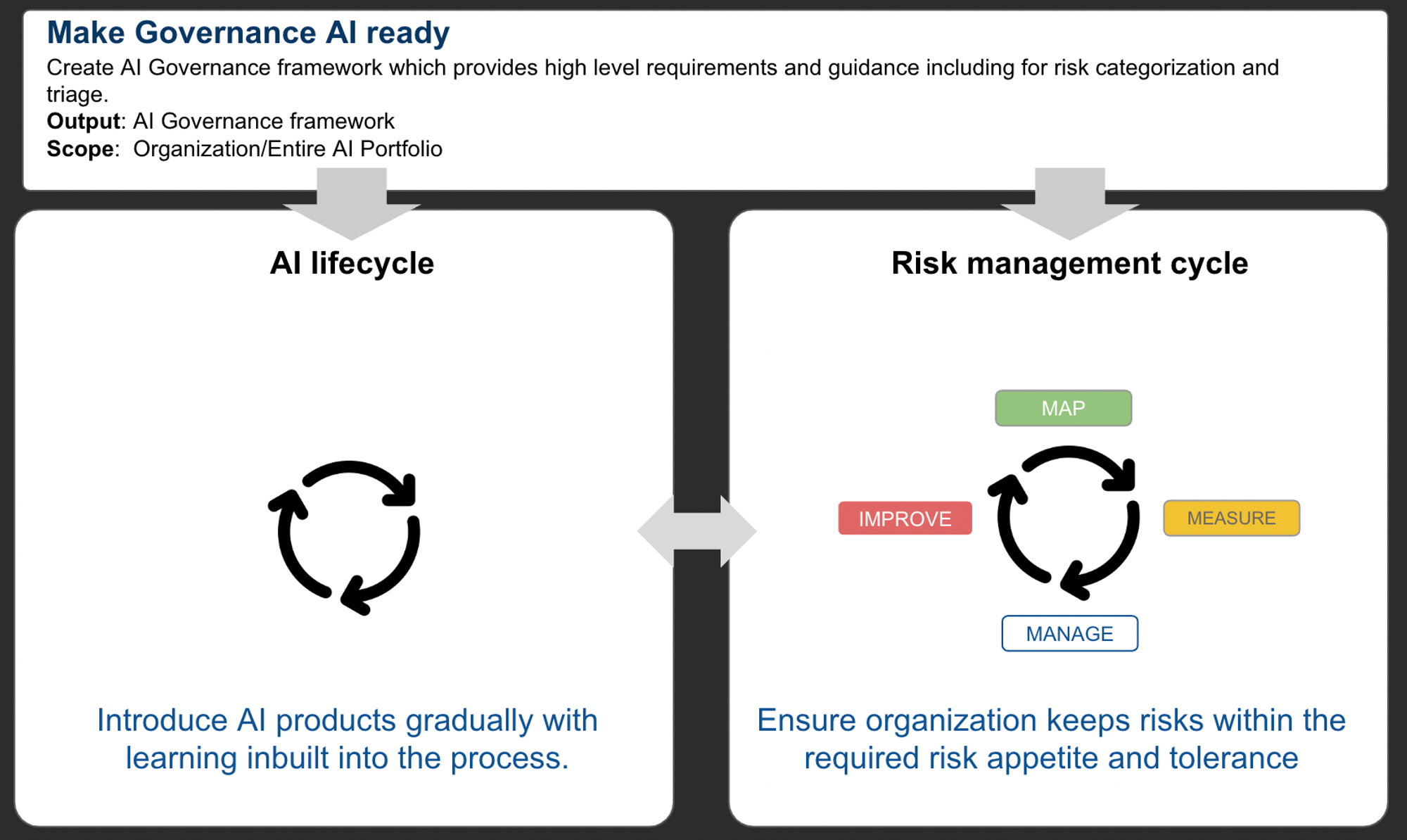
- Make Governance AI ready. This first tier fulfills organization wide policy objectives, ensures consistency and alignment with other frameworks and standards and provides high level guidance and prescriptions. Guidelines for application level risk categorization and triage will be part of this tier.
- MAP function which operationalizes the framework and is part of the risk management lifecycle. This second tier incorporates essential use case specific information which finally helps complete the picture. At this stage, triage and application risk categorization are performed, guiding the subsequent workstreams based on the assigned risk classification.
Scalability
Both risk and business units should have a common aim of ensuring core business functions of the organization are not affected while at the same time ensuring AI risks are kept within tolerance. While the previous horizontal tiering mechanism gave the underlying capability to the organization, “vertical” tiering via a triage process will permit it to address scale.
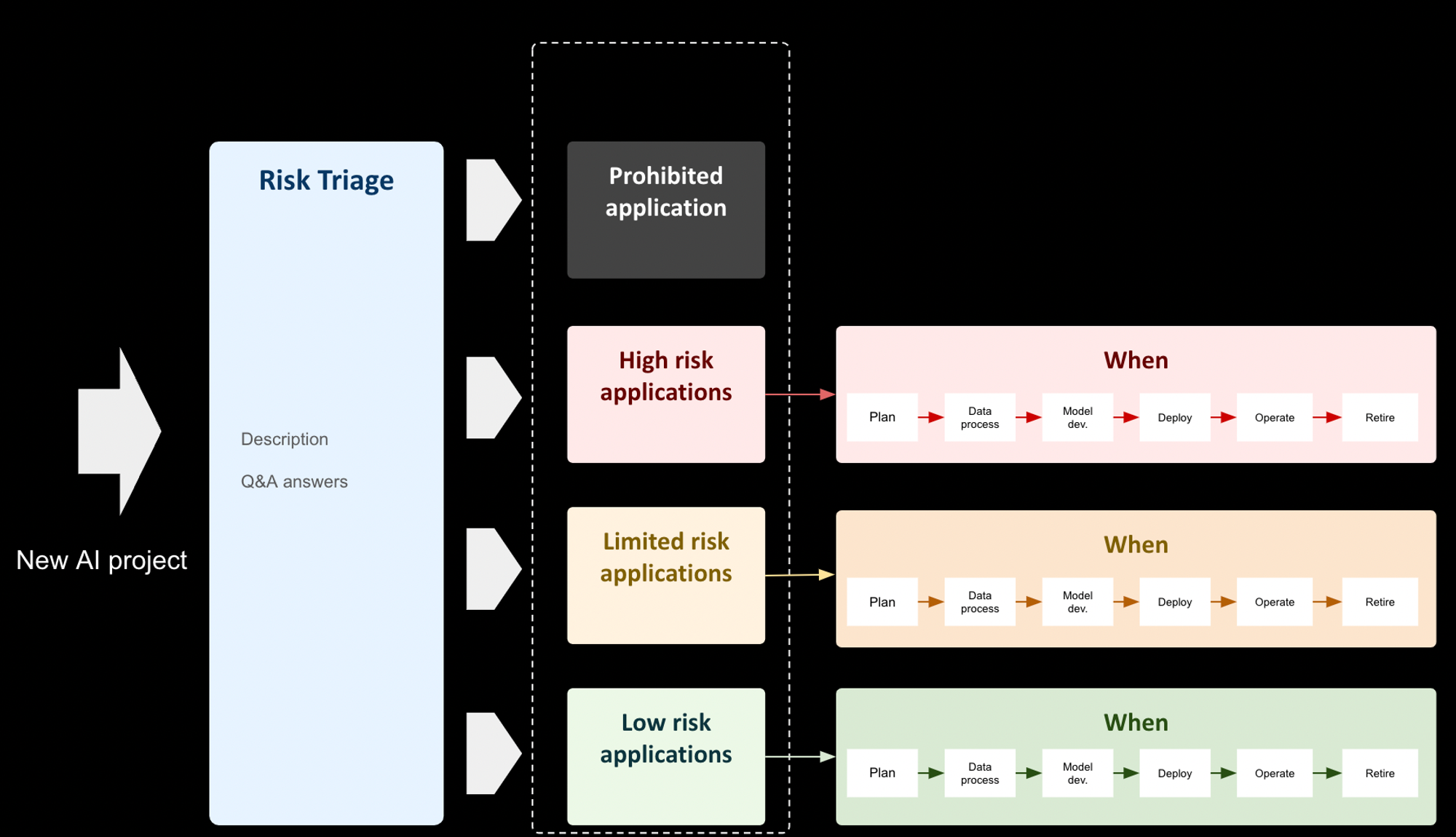
The application level risk categorization, while aligned with the emerging regulatory landscape, is illustrative and in general a more fit-for-purpose tiering needs to be created. Service levels can be set with escalations built-in, specially for the low and limited risk tiers, to ensure processes do not become bureaucratic and to support business planning.
Takeaways
Increasingly ubiquitous nature of AI applications, fast paced change in technology, shorter risk management cycles and tiered regulatory obligations - the consensus is that a one size fits all approach to AI governance is not practical for the vast majority of organizations.
- The tradeoffs between competing objectives are also real - approaches which are comprehensive, if not designed properly, can affect the scalability and flexibility of the programs rendering them too governance heavy and stifling innovation - and while they can be mitigated with proper understanding of the system and smarter approaches, they cannot be eliminated. This is specially true of organizations in regulated sectors that need to exercise greater caution.
- Approaches need to align with the emerging regulatory landscape in order to minimize future regulatory and legal risks.
- A tiered approach to risk management, as proposed here, can help better balance the tradeoffs in a more granular and sustainable manner. This also aligns with the emerging regulatory trends.
Having said all this, nothing beats a solid culture which requires stakeholders to understand and appreciate both AI innovation and risk management.
References
NIST AI RMF 2023: https://www.nist.gov/itl/ai-risk-management-framework
About the EU AI Act: https://www.europarl.europa.eu/topics/en/article/20230601STO93804/eu-ai-act-first-regulation-on-artificial-intelligence